Paper in IJCARS (2016) on “Automated video-based assessment of surgical skills for training and evaluation in medical schools”
Paper
Automated video-based assessment of surgical skills for training and evaluation in medical schools Journal Article
In: International Journal of Computer Assisted Radiology and Surgery, vol. 11, no. 9, pp. 1623–1636, 2016.
Abstract
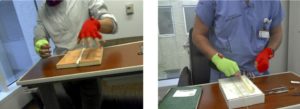
Purpose: Routine evaluation of basic surgical skills in medical schools requires considerable time and effort from supervising faculty. For each surgical trainee, a supervisor has to observe the trainees in-person. Alternatively, supervisors may use training videos, which reduces some of the logistical overhead. All these approaches, however, are still incredibly time-consuming and involve human bias. In this paper, we present an automated system for surgical skills assessment by analyzing video data of surgical activities.
Method : We compare different techniques for video-based surgical skill evaluation. We use techniques that capture the motion information at a coarser granularity using symbols or words, extract motion dynamics using textural patterns in a frame kernel matrix, and analyze fine-grained motion information using frequency analysis. Results: We were successfully able to classify surgeons into different skill levels with high accuracy. Our results indicate that fine-grained analysis of motion dynamics via frequency analysis is most effective in capturing the skill-relevant information in surgical videos.
Conclusion: Our evaluations show that frequency features perform better than motion texture features, which in turn perform better than symbol/word-based features. Put succinctly, skill classification accuracy is positively correlated with motion granularity as demonstrated by our results on two challenging video datasets.